Approach
Tech-centered research / design
Key Skills
ML Training
Research
UI/UX
Prototyping
Duration
14 weeks
Tools
Figma
Runway
Photoshop
After Effects
LUMIS
This platform is a space where subject matter experts can contribute images to build more unbiased datasets used for training AI and Machine Learning models.
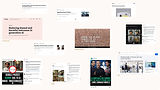
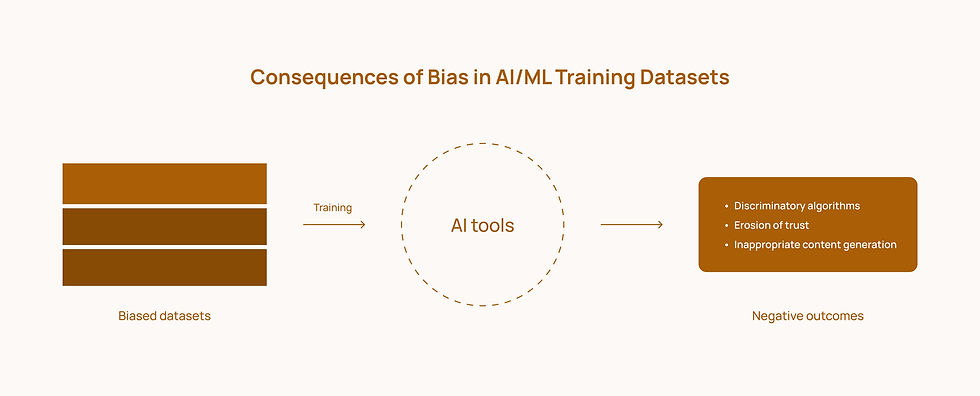
Problems
Bias in AI/ML training datasets & reliance on small, select groups of hired workers for image labeling, with unclear criteria for how they are chosen.
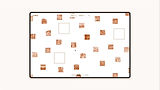

My Approach
Instead of relying on a select few who may not be familiar with all categories, our platform allows subject matter experts to contribute directly to image data collection and labeling processes.
This publicly monitored environment ensures accurate and appropriate data and labels, resulting in higher-quality image datasets.

Evolving Datasets
This platform initially utilizes existing image datasets, such as 'ImageNet' as a base. Through the feedback loop of uploading images and validating them, it evolves and improves over time.
Business Model
This platform aims to sell the improved datasets to companies for AI/ML training. The strength lies in offering diverse aspect, which enhances AI performance by reducing bias.
Home Page Design
This UI presents images in a floating arrangement, implying that they are randomly displayed based on users’ expertise.

Diverse Dataset Expansion
In this community-driven platform, subject matter experts upload images to expand underrepresented data within various categories.
This approach ensures those familiar with specific categories guide dataset growth.

LUMIS ensures accurate and culturally nuanced image categorization by involving diverse global experts in the class and label validation process.
Democratizing Data
Curation Process
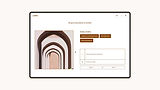
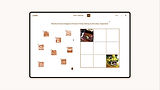
To increase users’ engagement and provide additional incentives, I gamified the experience of validating classes and labels.
Gamified Experience
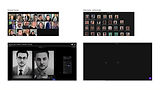
Problem Discovery
I trained a model on Runway using my selfies to generate AI photos, but unlike the tutorial's accurate results, mine didn’t resemble me. This led me to question if model performance varies across different racial groups.
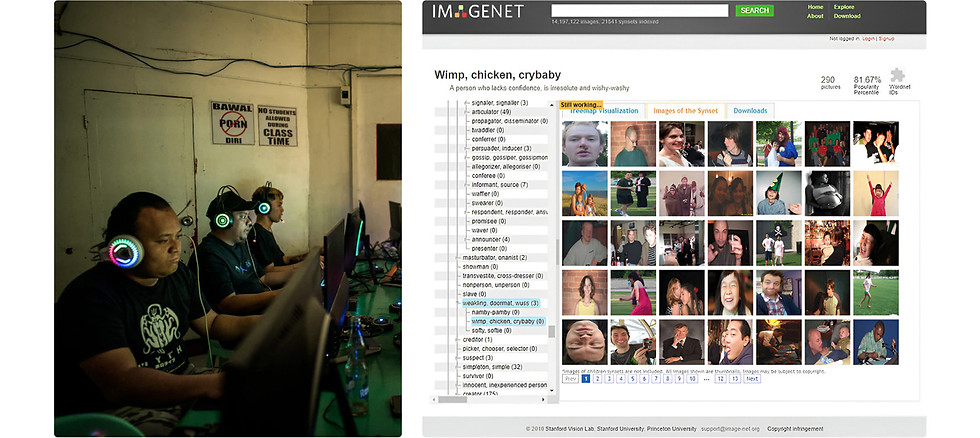
Desk Research
- In the current AI industry, companies often depend on a certain group of individuals for data labeling such as people in Africa & Philippines for low wages.
- Secondly, a lot of the current AI tools are trained on a datasets called “ImageNet”, which has many inappropriate labels.

Typography
I chose the typeface, ‘Sora’ for its blend of neutrality and uniqueness. Its distinct yet approachable style effectively represents the variety and inclusivity.
Color
I chose warm tone colors to give the datasets a more human and warm feel. Consistently using this color scheme throughout the platform underscores our collective effort and strengthens the sense of community.
Wireframing
After exploring hierarchical layouts for usability, user feedback showed that the platform's core concept—highlighting missing data types—was unclear. This led me to shift to more exploratory layouts, using empty space to better convey the message visually.

Other projects
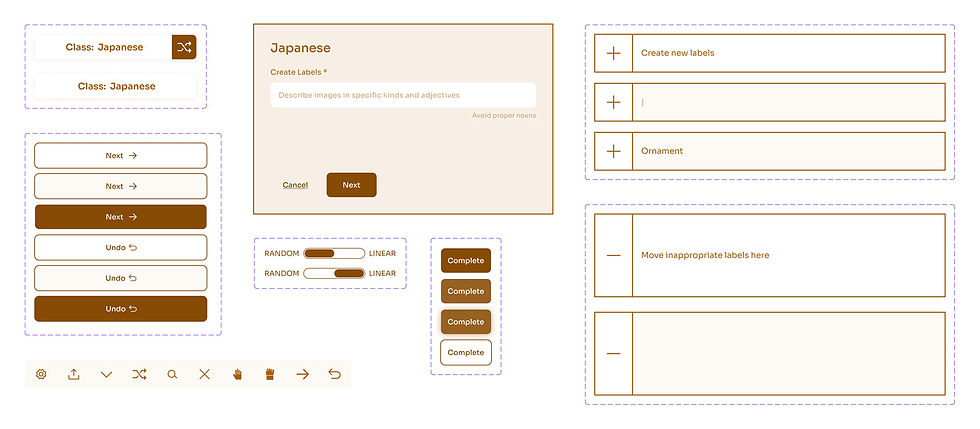
Working on this project marked my first experience with a tech-centered approach—creating concepts by directly experimenting with technology rather than initiating with user research. I'm particularly proud to have identified a significant problem in the AI space and to have developed a unique system and visual style from scratch. Additionally, this project allowed me to focus on micro-interactions and refine small UI components, such as filter chips for search, which I hadn't been able to delve into in previous projects.
Next Steps
-
Develop a reward-based ecosystem: Implement an incentive system that rewards user contribution, encouraging ongoing participation and engagement.
-
Interview passionate users: Conduct interviews to identify key traits for selecting the initial group of users.
-
Launch beta and set metrics: Release the beta version and track key metrics like active users and image uploads / validations to establish success benchmarks.

Reflection
